Measuring the emotional charge: Analysis of the emotions present in the content of tweets about COVID-19 in Lima
DOI:
https://doi.org/10.51252/rcsi.v3i2.587Keywords:
BERT, BETO, emotions, covid-19, PLNAbstract
During the state of emergency and quarantines implemented by world leaders, there has been a significant increase in people's activity on social networks, such as Twitter, where they share opinions and emotionally charged news. In this study, we present a visualization tool for sentiment analysis in tweets related to COVID-19 in the city of Lima, Peru, during the year 2020. For this purpose, we train a BERT model called BETO, specifically designed for natural language processing in Spanish. We used the SenWave dataset, comprising 11 emotions, to train the model. Subsequently, we validate the model using a dataset composed of 33,770 tweets collected in the city of Lima, Peru. The result of our study is an interactive dashboard showing the flow of sentiments expressed in the analyzed tweets. Our findings reveal that the three most frequent emotions during 2020 were: humor, boredom and optimism. In addition, we identified the five most popular words used in the tweets: contagion, health, distancing, isolation and Martín Vizcarra, referring to the former president of Peru.
References
Alturayeif, N., & Luqman, H. (2021). Fine-Grained Sentiment Analysis of Arabic COVID-19 Tweets Using BERT-Based Transformers and Dynamically Weighted Loss Function. Applied Sciences, 11(22), 10694. https://doi.org/10.3390/app112210694
Aygun, I., Kaya, B., & Kaya, M. (2022). Aspect Based Twitter Sentiment Analysis on Vaccination and Vaccine Types in COVID-19 Pandemic With Deep Learning. IEEE Journal of Biomedical and Health Informatics, 26(5), 2360–2369. https://doi.org/10.1109/JBHI.2021.3133103
Blanco, G., & Lourenço, A. (2022). Optimism and pessimism analysis using deep learning on COVID-19 related twitter conversations. Information Processing & Management, 59(3), 102918. https://doi.org/10.1016/j.ipm.2022.102918
Cañete, J., Chaperon, G., Fuentes, R., Ho, J.-H., Kang, H., &, & Pérez, J. (2020). Spanish Pre-Trained BERT Model and Evaluation Data. PML4DC at ICLR. https://github.com/dccuchile/beto
Caraballo Ayala, N. E., Carreño Miranda, R., & Paternina Salgado, V. A. (2021). Análisis de sentimientos en Twitter: Opiniones en Colombia de los Juegos Olímpicos 2021. Uniwersytet Śląski. http://hdl.handle.net/10584/9874
Garcia, K., & Berton, L. (2021). Topic detection and sentiment analysis in Twitter content related to COVID-19 from Brazil and the USA. Applied Soft Computing, 101, 107057. https://doi.org/10.1016/j.asoc.2020.107057
Imvimol, C., & Chongstitvatana, P. (2021). Sentiment analysis of messages on Twitter related to COVID-19 using deep learning approach. 2021 25th International Computer Science and Engineering Conference (ICSEC), 363–367. https://doi.org/10.1109/ICSEC53205.2021.9684587
IPSOS. (2020). Uso de Redes Sociales entre peruanos conectados 2020. Institut de Publique Sondage d’Opinion Secteur. https://www.ipsos.com/es-pe/uso-de-redes-sociales-entre-peruanos-conectados-2020
Mendoza Castillo, L. (1970). Lo que la pandemia nos enseñó sobre la educación a distancia. Revista Latinoamericana de Estudios Educativos, 50(ESPECIAL), 343–352. https://doi.org/10.48102/rlee.2020.50.ESPECIAL.119
Mohamed Ridhwan, K., & Hargreaves, C. A. (2021). Leveraging Twitter data to understand public sentiment for the COVID‐19 outbreak in Singapore. International Journal of Information Management Data Insights, 1(2), 100021. https://doi.org/10.1016/j.jjimei.2021.100021
Sitaula, C., Basnet, A., Mainali, A., & Shahi, T. B. (2021). Deep Learning-Based Methods for Sentiment Analysis on Nepali COVID-19-Related Tweets. Computational Intelligence and Neuroscience, 2021, 1–11. https://doi.org/10.1155/2021/2158184
Topbas, A., Jamil, A., Hameed, A. A., Ali, S. M., Bazai, S., & Shah, S. A. (2021). Sentiment Analysis for COVID-19 Tweets Using Recurrent Neural Network (RNN) and Bidirectional Encoder Representations (BERT) Models. 2021 International Conference on Computing, Electronic and Electrical Engineering (ICE Cube), 1–6. https://doi.org/10.1109/ICECube53880.2021.9628315
Wankhade, M., & Rao, A. C. S. (2022). Opinion analysis and aspect understanding during covid-19 pandemic using BERT-Bi-LSTM ensemble method. Scientific Reports, 12(1), 17095. https://doi.org/10.1038/s41598-022-21604-7
Yang, Q., Alamro, H., Albaradei, S., Salhi, A., Lv, X., Ma, C., Alshehri, M., Jaber, I., Tifratene, F., Wang, W., Gojobori, T., Duarte, C. M., Gao, X., & Zhang, X. (2020). SenWave: Monitoring the Global Sentiments under the COVID-19 Pandemic. http://arxiv.org/abs/2006.10842
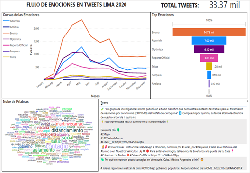
Published
How to Cite
Issue
Section
License
Copyright (c) 2023 Luis Alberto Holgado-Apaza, Coren Luhana Ancco-Calloapaza, Octavio Bedregal-Flores, Marleny Quispe-Layme, Ralph Miranda-Castillo

This work is licensed under a Creative Commons Attribution 4.0 International License.
The authors retain their rights:
a. The authors retain their trademark and patent rights, as well as any process or procedure described in the article.
b. The authors retain the right to share, copy, distribute, execute and publicly communicate the article published in the Revista Científica de Sistemas e Informática (RCSI) (for example, place it in an institutional repository or publish it in a book), with an acknowledgment of its initial publication in the RCSI.
c. Authors retain the right to make a subsequent publication of their work, to use the article or any part of it (for example: a compilation of their works, notes for conferences, thesis, or for a book), provided that they indicate the source of publication (authors of the work, journal, volume, number and date).