Sentiment analysis in social network X for the evaluation of the positioning of candidates in political elections
DOI:
https://doi.org/10.51252/rcsi.v5i1.763Keywords:
KDD Methodology, Data Mining, NLP, Sentiment PolarityAbstract
Social networks are among the most important means of political communication, through which opinions are published and generated on various topics; for this reason, they are an excellent means to analyze and understand events. In this work, sentiment analysis of X/Twitter posts about the elections of presidential candidates in Mexico in 2024 was carried out via the classification of sentiment polarity to measure the positioning of the participants. A methodology based on KDD was used in this research, and 151,821 posts were analyzed about four aspiring candidates for the presidency of Mexico from the MORENA party. The results showed that the candidates best positioned in the election obtained the highest number of posts with positive polarity, although the winning candidate did not coincide with the highest percentage of positive polarities. This result indicates that it is necessary to include other variables in addition to polarity to more accurately predict the winners of political contests.
References
Aljabri, M., Zagrouba, R., Shaahid, A., Alnasser, F., Saleh, A., & Alomari, D. M. (2023). Machine learning-based social media bot detection: a comprehensive literature review. Social Network Analysis and Mining, 13(1), 20. https://doi.org/10.1007/s13278-022-01020-5 DOI: https://doi.org/10.1007/s13278-022-01020-5
Ansari, M. Z., Aziz, M. B., Siddiqui, M. O., Mehra, H., & Singh, K. P. (2020). Analysis of Political Sentiment Orientations on Twitter. Procedia Computer Science, 167, 1821-1828. https://doi.org/10.1016/j.procs.2020.03.201 DOI: https://doi.org/10.1016/j.procs.2020.03.201
Antonakaki, D., Fragopoulou, P., & Ioannidis, S. (2021). A survey of Twitter research: Data model, graph structure, sentiment analysis and attacks. Expert Systems with Applications, 164, 114006. https://doi.org/10.1016/j.eswa.2020.114006 DOI: https://doi.org/10.1016/j.eswa.2020.114006
Bharany, S., Alam, S., Shuaib, M., & Talwar, B. (2023). Sentiment Analysis of Twitter Data for COVID-19 Posts (pp. 457-466). https://doi.org/10.1007/978-981-19-6004-8_37 DOI: https://doi.org/10.1007/978-981-19-6004-8_37
Birjali, M., Kasri, M., & Beni-Hssane, A. (2021). A comprehensive survey on sentiment analysis: Approaches, challenges and trends. Knowledge-Based Systems, 226, 107134. https://doi.org/10.1016/j.knosys.2021.107134 DOI: https://doi.org/10.1016/j.knosys.2021.107134
Caetano, J. A., Lima, H. S., Santos, M. F., & Marques-Neto, H. T. (2018). Using sentiment analysis to define twitter political users’ classes and their homophily during the 2016 American presidential election. Journal of Internet Services and Applications, 9(1), 18. https://doi.org/10.1186/s13174-018-0089-0 DOI: https://doi.org/10.1186/s13174-018-0089-0
Cantini, R., Marozzo, F., Talia, D., & Trunfio, P. (2022). Analyzing Political Polarization on Social Media by Deleting Bot Spamming. Big Data and Cognitive Computing, 6(1), 3. https://doi.org/10.3390/bdcc6010003 DOI: https://doi.org/10.3390/bdcc6010003
Chaudhry, H. N., Javed, Y., Kulsoom, F., Mehmood, Z., Khan, Z. I., Shoaib, U., & Janjua, S. H. (2021). Sentiment Analysis of before and after Elections: Twitter Data of U.S. Election 2020. Electronics, 10(17), 2082. https://doi.org/10.3390/electronics10172082 DOI: https://doi.org/10.3390/electronics10172082
Cheng, C., Luo, Y., & Yu, C. (2020). Dynamic mechanism of social bots interfering with public opinion in network. Physica A: Statistical Mechanics and its Applications, 551, 124163. https://doi.org/10.1016/j.physa.2020.124163 DOI: https://doi.org/10.1016/j.physa.2020.124163
Debuse, J. C. W., de la Iglesia, B., Howard, C. M., & Rayward-Smith, V. J. (2001). Building the KDD Roadmap. En Industrial Knowledge Management (pp. 179-196). Springer London. https://doi.org/10.1007/978-1-4471-0351-6_12 DOI: https://doi.org/10.1007/978-1-4471-0351-6_12
Espejel Espinoza, A., & Díaz Sandoval, M. (2022). Tendencias organizacionales y democracia interna en los partidos políticos en México. Los casos del PAN, PRI, PRD, PT, PVEM, MC y MORENA (1.a ed.). Facultad de Estudios Superiores Acatlán, UNAM.
Flamino, J., Galeazzi, A., Feldman, S., Macy, M. W., Cross, B., Zhou, Z., Serafino, M., Bovet, A., Makse, H. A., & Szymanski, B. K. (2023). Political polarization of news media and influencers on Twitter in the 2016 and 2020 US presidential elections. Nature Human Behaviour. https://doi.org/10.1038/s41562-023-01550-8 DOI: https://doi.org/10.1038/s41562-023-01550-8
Garg, P. K., Pandey, M., & Arora, M. (2020). Sentiment Analysis for Predicting the Popularity of Web Series. En Communications in Computer and Information Science (1.a ed., pp. 133-140). Springer Nature. https://doi.org/10.1007/978-981-15-5830-6_12 DOI: https://doi.org/10.1007/978-981-15-5830-6_12
Gilardi, F., Gessler, T., Kubli, M., & Müller, S. (2022). Social Media and Political Agenda Setting. Political Communication, 39(1), 39-60. https://doi.org/10.1080/10584609.2021.1910390 DOI: https://doi.org/10.1080/10584609.2021.1910390
Lucca, J. B. (2019). Teoría y política en la génesis de MORENA como nuevo partido. Estudios Políticos, 49. https://doi.org/10.22201/fcpys.24484903e.2020.49.72396 DOI: https://doi.org/10.22201/fcpys.24484903e.2020.49.72396
Marín Dueñas, P. P., Simancas González, E., & Berzosa Moreno, A. (2019). Uso e influencia de Twitter en la comunicación política: el caso del Partido Popular y Podemos en las elecciones generales de 2016. Cuadernos.info, 45, 129-144. https://doi.org/10.7764/cdi.45.1595 DOI: https://doi.org/10.7764/cdi.45.1595
Noor, H. M., Turetken, O., & Akgul, M. (2024). Social Media, Sentiments and Political Discourse – An Exploratory Study of the 2021 Canadian Federal Election. ACM Transactions on Social Computing, 7(1-4), 1-23. https://doi.org/10.1145/3665450 DOI: https://doi.org/10.1145/3665450
Oliveira, D. J. S., Bermejo, P. H. de S., Pereira, J. R., & Barbosa, D. A. (2019). The application of the sentiment analysis technique in social media as a tool for social management practices at the governmental level. Revista de Administração Pública, 53(1), 235-251. https://doi.org/10.1590/0034-7612174204 DOI: https://doi.org/10.1590/0034-7612174204
Ongo Nkoa, B. E., Ondoua Beyene, B., Ngo Nsoa Simb, J. F., & Ngnouwal Eloundou, G. (2023). Does social media improve women’s political empowerment in Africa? Telecommunications Policy, 47(9), 102624. https://doi.org/10.1016/j.telpol.2023.102624 DOI: https://doi.org/10.1016/j.telpol.2023.102624
Oueslati, O., Hajhmida, M. Ben, Ounelli, H., & Cambria, E. (2023). Sentiment Analysis of Influential Messages for Political Election Forecasting. En International Conference on Computational Linguistics and Intelligent Text Processing (1.a ed., pp. 280-292). La Rochelle. https://doi.org/10.1007/978-3-031-24340-0_21 DOI: https://doi.org/10.1007/978-3-031-24340-0_21
Russo, R., Blikstein, P., & Literat, I. (2024). Twisted knowledge construction on X/Twitter: an analysis of constructivist sensemaking on social media leading to political radicalization. Information and Learning Sciences, 125(9), 693-719. https://doi.org/10.1108/ILS-12-2023-0210 DOI: https://doi.org/10.1108/ILS-12-2023-0210
Sarapugdi, W., & Namkhun, S. (2023). A Social Analysis of Thailand’s 2023 Election Through Twitter Feeds. 2023 15th International Conference on Information Technology and Electrical Engineering (ICITEE), 208-212. https://doi.org/10.1109/ICITEE59582.2023.10317682 DOI: https://doi.org/10.1109/ICITEE59582.2023.10317682
Sobkowicz, A., & Kozłowski, M. (2018). Sentiment Analysis in Polish Web-Political Discussions. En Human Language Technology. Challenges for Computer Science and Linguistics (pp. 363-377). Springer Nature. https://doi.org/10.1007/978-3-319-93782-3_26 DOI: https://doi.org/10.1007/978-3-319-93782-3_26
Turón, A., Altuzarra, A., Moreno-Jiménez, J. M., & Navarro, J. (2023). Evolution of social mood in Spain throughout the COVID-19 vaccination process: a machine learning approach to tweets analysis. Public Health, 215, 83-90. https://doi.org/10.1016/j.puhe.2022.12.003 DOI: https://doi.org/10.1016/j.puhe.2022.12.003
Vyas, V., & Uma, V. (2018). An Extensive study of Sentiment Analysis tools and Binary Classification of tweets using Rapid Miner. Procedia Computer Science, 125, 329-335. https://doi.org/10.1016/j.procs.2017.12.044 DOI: https://doi.org/10.1016/j.procs.2017.12.044
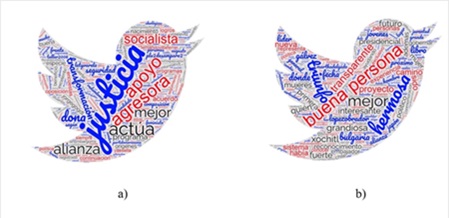
Downloads
Published
How to Cite
Issue
Section
License
Copyright (c) 2025 María Claudia Denicia-Carral, Ana Luisa Ballinas-Hernández, Gustavo Manuel Minquiz-Xolo, Héctor Medina-Cruz

This work is licensed under a Creative Commons Attribution 4.0 International License.
The authors retain their rights:
a. The authors retain their trademark and patent rights, as well as any process or procedure described in the article.
b. The authors retain the right to share, copy, distribute, execute and publicly communicate the article published in the Revista Científica de Sistemas e Informática (RCSI) (for example, place it in an institutional repository or publish it in a book), with an acknowledgment of its initial publication in the RCSI.
c. Authors retain the right to make a subsequent publication of their work, to use the article or any part of it (for example: a compilation of their works, notes for conferences, thesis, or for a book), provided that they indicate the source of publication (authors of the work, journal, volume, number and date).