Intelligent optimization of rural water infrastructure through big data and predictive models: Insights from Latin America
DOI:
https://doi.org/10.51252/rcsi.v5i1.762Keywords:
Algorithms, Digitalization, Monitoring, Supply, SustainabilityAbstract
Access to potable water in rural areas continues to face structural challenges due to technological, operational, and planning gaps. In this study, we reviewed the state of the art on the use of Big Data and Artificial Intelligence for optimizing rural water infrastructure. We conducted a systematic review using the Scopus database, covering publications from 2015 to 2025. We identified 582 articles, of which 48 met the inclusion criteria. Our results show that predictive models and massive data analysis have improved operational efficiency by anticipating failures in distribution networks with up to 85% accuracy, thereby reducing losses. Additionally, technologies such as IoT sensors, digital twins, and automated systems have been successfully implemented in various countries, generating positive impacts on service sustainability. We conclude that the digitalization of potable water management, through AI and Big Data, constitutes an effective strategy to enhance the resilience and quality of supply in rural settings. These findings provide key inputs for designing policies and technological solutions applicable to regions such as San Martín, Peru.
References
Abbas, F., Cai, Z., Shoaib, M., Iqbal, J., Ismail, M., Arifullah, Alrefaei, A. F., & Albeshr, M. F. (2024). Machine Learning Models for Water Quality Prediction: A Comprehensive Analysis and Uncertainty Assessment in Mirpurkhas, Sindh, Pakistan. Water, 16(7), 941. https://doi.org/10.3390/w16070941 DOI: https://doi.org/10.3390/w16070941
Abushandi, E. (2025). Water Quality Assessment and Forecasting Along the Liffey and Andarax Rivers by Artificial Neural Network Techniques Toward Sustainable Water Resources Management. Water, 17(3), 453. https://doi.org/10.3390/w17030453 DOI: https://doi.org/10.3390/w17030453
Ahmed, A. A., Sayed, S., Abdoulhalik, A., Moutari, S., & Oyedele, L. (2024). Applications of machine learning to water resources management: A review of present status and future opportunities. Journal of Cleaner Production, 441, 140715. https://doi.org/10.1016/j.jclepro.2024.140715 DOI: https://doi.org/10.1016/j.jclepro.2024.140715
Ainapure, B., Baheti, N., Buch, J., Appasani, B., Jha, A. V., & Srinivasulu, A. (2023). Drinking water potability prediction using machine learning approaches: a case study of Indian rivers. Water Practice & Technology, 18(12), 3004–3020. https://doi.org/10.2166/wpt.2023.202 DOI: https://doi.org/10.2166/wpt.2023.202
Alselek, M., Alcaraz-Calero, J. M., Segura-Garcia, J., & Wang, Q. (2022). Water IoT Monitoring System for Aquaponics Health and Fishery Applications. Sensors, 22(19). https://doi.org/10.3390/S22197679 DOI: https://doi.org/10.3390/s22197679
Amini, M., & Dziedzic, R. (2022). Comparison of Machine Learning Classifiers for Predicting Water Main Failure (pp. 501–512). https://doi.org/10.1007/978-981-19-1065-4_42 DOI: https://doi.org/10.1007/978-981-19-1065-4_42
Ao, Y., Fan, J., Guo, F., Li, M., Li, A., Shi, Y., & Wei, J. (2025). Machine Learning-Based Early Warning of Algal Blooms: A Case Study of Key Environmental Factors in the Anzhaoxin River Basin. Water, 17(5), 725. https://doi.org/10.3390/w17050725 DOI: https://doi.org/10.3390/w17050725
Ashraf, E., Kabeel, A. E., Elmashad, Y., Ward, S. A., & Shaban, W. M. (2023). Predicting solar distiller productivity using an AI Approach: Modified genetic algorithm with Multi-Layer Perceptron. Solar Energy, 263, 111964. https://doi.org/10.1016/j.solener.2023.111964 DOI: https://doi.org/10.1016/j.solener.2023.111964
Bonilla, C. A., Zanfei, A., Brentan, B., Montalvo, I., & Izquierdo, J. (2022). A Digital Twin of a Water Distribution System by Using Graph Convolutional Networks for Pump Speed-Based State Estimation. Water, 14(4), 514. https://doi.org/10.3390/w14040514 DOI: https://doi.org/10.3390/w14040514
Canahuire-Robles, R., Hilares-Vargas, S., & Peña-Valdiglesias, J. (2023). Influencia de las actividades antropogénicas en la calidad del agua de la microcuenca Chonta en la provincia Tambopata, Madre de Dios. Revista Biodiversidad Amazónica, 2(2), 54–69. https://doi.org/10.55873/rba.v2i2.254 DOI: https://doi.org/10.55873/rba.v2i2.254
Cheng, Y.-M., Horng, M.-F., & Chung, C.-C. (2024). ICT Innovation to Promote Sustainable Development Goals: Implementation of Smart Water Pipeline Monitoring System Based on Narrowband Internet of Things. Sustainability, 16(22), 9683. https://doi.org/10.3390/su16229683 DOI: https://doi.org/10.3390/su16229683
Chowdhury, S., & Karanfil, T. (2024). Applications of artificial intelligence (AI) in drinking water treatment processes: Possibilities. Chemosphere, 356, 141958. https://doi.org/10.1016/j.chemosphere.2024.141958 DOI: https://doi.org/10.1016/j.chemosphere.2024.141958
Da Silva, S., Britto, V., Azevedo, C., & Kiperstok, A. (2014). Rational Consumption of Water in Administrative Public Buildings: The Experience of the Bahia Administrative Center, Brazil. Water, 6(9), 2552–2574. https://doi.org/10.3390/w6092552 DOI: https://doi.org/10.3390/w6092552
de Almeida, F. C. L., Brennan, M. J., Iwanaga, M. K., de Oliveira Cézar, S., Bispo, L. A. F., & Scussel, O. (2025). A Web-Based Simulator for Leak Detection Problems in Water Distribution Pipes Using Vibro-Acoustic Techniques (pp. 69–81). https://doi.org/10.1007/978-3-031-71540-2_6 DOI: https://doi.org/10.1007/978-3-031-71540-2_6
Del-Águila-Chávez, J., García-Meléndez, R. L., García-Ruiz, L., & Casado-del-Castillo, S. P. (2024). Diversidad de camarones de agua dulce (Crustacea: Palaemonidae) en afluentes de la cuenca alta del rio Itaya, Loreto, Perú. Revista Peruana de Investigación Agropecuaria, 3(2), e73. https://doi.org/10.56926/repia.v3i2.73 DOI: https://doi.org/10.56926/repia.v3i2.73
Dodanwala, T. C., & Ruparathna, R. (2024). A Levels of Service (LOS) Digital Twin for Potable Water Infrastructure Systems (pp. 15–37). https://doi.org/10.1007/978-3-031-61519-1_2 DOI: https://doi.org/10.1007/978-3-031-61519-1_2
Encinas, C., Ruiz, E., Cortez, J., & Espinoza, A. (2017). Design and implementation of a distributed IoT system for the monitoring of water quality in aquaculture. Wireless Telecommunications Symposium. https://doi.org/10.1109/WTS.2017.7943540 DOI: https://doi.org/10.1109/WTS.2017.7943540
Entezami, A., Behkamal, B., & De Michele, C. (2024). Long-Term Structural Health Monitoring by Remote Sensing and Advanced Machine Learning. Springer Nature Switzerland. https://doi.org/10.1007/978-3-031-53995-4 DOI: https://doi.org/10.1007/978-3-031-53995-4
Esquivel-Ayala, B. D., & Tapia-Cabrera, C. F. (2021). La importancia de la economía del agua. RD-ICUAP, 7(21), 78–91. http://rd.buap.mx/ojs-dm/index.php/rdicuap/article/view/630 DOI: https://doi.org/10.32399/icuap.rdic.2448-5829.2021.21.630
Eva, E. A., Marzen, L. J., & Lamba, J. S. (2024). Modeling the effect of LULC change on water quantity and quality in Big Creek Lake Watershed, South Alabama USA. The Egyptian Journal of Remote Sensing and Space Sciences, 27(2), 277–287. https://doi.org/10.1016/j.ejrs.2024.03.005 DOI: https://doi.org/10.1016/j.ejrs.2024.03.005
Fardan, S., & Al-Sartawi, A. (2023). Roll of Artificial Intelligence in Smart Metering System for Water Distribution (pp. 291–298). https://doi.org/10.1007/978-3-031-43300-9_24 DOI: https://doi.org/10.1007/978-3-031-43300-9_24
Fares, A., Tijani, I. A., Rui, Z., & Zayed, T. (2023). Leak detection in real water distribution networks based on acoustic emission and machine learning. Environmental Technology, 44(25), 3850–3866. https://doi.org/10.1080/09593330.2022.2074320 DOI: https://doi.org/10.1080/09593330.2022.2074320
García-Avila, F., Loja-Suco, P., Siguenza-Jeton, C., Jiménez-Ordoñez, M., Valdiviezo-Gonzales, L., Cabello-Torres, R., & Aviles-Añazco, A. (2023). Evaluation of the water quality of a high Andean lake using different quantitative approaches. Ecological Indicators, 154, 110924. https://doi.org/10.1016/j.ecolind.2023.110924 DOI: https://doi.org/10.1016/j.ecolind.2023.110924
García, G. A., García, P. E., Rovere, S. L., Bert, F. E., Schmidt, F., Menéndez, Á. N., Nosetto, M. D., Verdin, A., Rajagopalan, B., Arora, P., & Podestá, G. P. (2019). A linked modelling framework to explore interactions among climate, soil water, and land use decisions in the Argentine Pampas. Environmental Modelling & Software, 111, 459–471. https://doi.org/10.1016/j.envsoft.2018.10.013 DOI: https://doi.org/10.1016/j.envsoft.2018.10.013
Goodwill, J. E., Ray, P., Nock, D., & Miller, C. M. (2022). Emerging investigator series: moving beyond resilience by considering antifragility in potable water systems. Environmental Science: Water Research & Technology, 8(1), 8–21. https://doi.org/10.1039/D1EW00732G DOI: https://doi.org/10.1039/D1EW00732G
Hosny, K. M., El-Hady, W. M., & Samy, F. M. (2025). Technologies, Protocols, and applications of Internet of Things in greenhouse Farming: A survey of recent advances. Information Processing in Agriculture, 12(1), 91–111. https://doi.org/10.1016/j.inpa.2024.04.002 DOI: https://doi.org/10.1016/j.inpa.2024.04.002
Kimothi, S., Thapliyal, A., Akram, S. V., Singh, R., Gehlot, A., Mohamed, H. G., Anand, D., Ibrahim, M., & Noya, I. D. (2022). Big Data Analysis Framework for Water Quality Indicators with Assimilation of IoT and ML. Electronics, 11(13), 1927. https://doi.org/10.3390/electronics11131927 DOI: https://doi.org/10.3390/electronics11131927
Kumar, L. V. (2013). Business and urban water utility operations in India. Water and Energy International, 70(2), 40–44. https://www.scopus.com/inward/record.uri?eid=2-s2.0-84884397812&partnerID=40&md5=5b5dedd958238b0edf3a9e49e98855ba
Kyritsakas, G., Boxall, J. B., & Speight, V. L. (2023). A Big Data framework for actionable information to manage drinking water quality. AQUA — Water Infrastructure, Ecosystems and Society, 72(5), 701–720. https://doi.org/10.2166/aqua.2023.218 DOI: https://doi.org/10.2166/aqua.2023.218
Lahari, S. A., Kumawat, N., Amreen, K., Ponnalagu, R. N., & Goel, S. (2025). IoT integrated and deep learning assisted electrochemical sensor for multiplexed heavy metal sensing in water samples. Npj Clean Water, 8(1), 10. https://doi.org/10.1038/s41545-025-00441-x DOI: https://doi.org/10.1038/s41545-025-00441-x
Lenka, A. K. (2022). Inequalities in Access to Water Supply and Sanitation Facilities—A Study in Bhubaneswar City, Odisha. In Mapping Identity-Induced Marginalisation in India: Inclusion and Access in the Land of Unequal Opportunities (pp. 475–491). https://doi.org/10.1007/978-981-19-3128-4_26 DOI: https://doi.org/10.1007/978-981-19-3128-4_26
Martinez, G., McCord, S. A., Driscoll, C. T., Todorova, S., Wu, S., Araújo, J. F., Vega, C. M., & Fernandez, L. E. (2018). Mercury contamination in riverine sediments and fish associated with artisanal and small-scale gold mining in Madre de Dios, Peru. International Journal of Environmental Research and Public Health, 15(8). https://doi.org/10.3390/ijerph15081584 DOI: https://doi.org/10.3390/ijerph15081584
Masud Rana, S. M., Uber, J., McCary, J., & Keck, J. (2024). A Greedy Search Algorithm for Closed Valve Analysis in Drinking Water Networks for Real-Time Model Development. World Environmental and Water Resources Congress 2024, 1257–1264. https://doi.org/10.1061/9780784485477.112 DOI: https://doi.org/10.1061/9780784485477.112
Medina, J. D., Arias, A., Triana, J. M., Giraldo, L. F., SeguraQuijano, F., Gonzalez-Mancera, A., Zambrano, A. F., Quimbayo, J., & Castillo, E. (2022). Open-source low-cost design of a buoy for remote water quality monitoring in fish farming. PLoS ONE, 17(6 June). https://doi.org/10.1371/JOURNAL.PONE.0270202 DOI: https://doi.org/10.1371/journal.pone.0270202
Mohd Zebaral Hoque, J., Ab. Aziz, N. A., Alelyani, S., Mohana, M., & Hosain, M. (2022). Improving Water Quality Index Prediction Using Regression Learning Models. International Journal of Environmental Research and Public Health, 19(20), 13702. https://doi.org/10.3390/ijerph192013702 DOI: https://doi.org/10.3390/ijerph192013702
Nagar, U. P., & Patel, H. M. (2023). Development of Short-Term Reservoir Level Forecasting Models: A Case Study of Ajwa-Pratappura Reservoir System of Vishwamitri River Basin of Central Gujarat (pp. 261–269). https://doi.org/10.1007/978-981-19-9147-9_20 DOI: https://doi.org/10.1007/978-981-19-9147-9_20
Nemade, B., Maharana, K. K., Kulkarni, V., Mondal, S., Ghantasala, G. S. P., Al-Rasheed, A., Getahun, M., & Soufiene, B. O. (2024). IoT-based automated system for water-related disease prediction. Scientific Reports, 14(1), 29483. https://doi.org/10.1038/s41598-024-79989-6 DOI: https://doi.org/10.1038/s41598-024-79989-6
Orlov, V., Kukartsev, A., Panfilov, I., Kozlova, A., & Boyko, A. (2024). Machine learning in environmental monitoring: The case of water potability prediction. BIO Web of Conferences, 130, 03016. https://doi.org/10.1051/bioconf/202413003016 DOI: https://doi.org/10.1051/bioconf/202413003016
Pandey, J., & Verma, S. (2022). Water Quality Analysis and Prediction Techniques Using Artificial Intelligence (pp. 279–290). https://doi.org/10.1007/978-981-16-4177-0_29 DOI: https://doi.org/10.1007/978-981-16-4177-0_29
Pandey, S., & Mishra, S. (2025). A review of sensing technologies for arsenic detection in drinking water. International Journal of Environmental Science and Technology, 22(4), 2809–2832. https://doi.org/10.1007/s13762-024-05912-1 DOI: https://doi.org/10.1007/s13762-024-05912-1
Pappu, S., Vudatha, P., Niharika, A. V, Karthick, T., & Sankaranarayanan, S. (2017). Intelligent IoT based water quality monitoring system. International Journal of Applied Engineering Research, 12(16), 5447–5454. https://www.scopus.com/inward/record.uri?eid=2-s2.0-85047172089&partnerID=40&md5=1f9af305e8dac25697a9488e980966d0
Peralta-Mahecha, G., Alarcón-García, S. J., Garzón-Camacho, J. C., Neuta-Niño, D. F., & Rodríguez-Arregocés, N. X. (2021). Desabastecimiento hídrico en el sistema de ciudades de Colombia: ordenamiento ambiental y territorial en el Área Hidrográfica Magdalena-Cauca. Cuadernos de Geografía: Revista Colombiana de Geografía, 30(2), 459–480. https://doi.org/10.15446/rcdg.v30n2.88753 DOI: https://doi.org/10.15446/rcdg.v30n2.88753
Pérez-Padillo, J., Morillo, J. G., Poyato, E. C., & Montesinos, P. (2021). Open-Source Application for Water Supply System Management: Implementation in a Water Transmission System in Southern Spain. Water, 13(24), 3652. https://doi.org/10.3390/w13243652 DOI: https://doi.org/10.3390/w13243652
Pesantez, J. E., Alghamdi, F., Sabu, S., Mahinthakumar, G., & Berglund, E. Z. (2022). Using a digital twin to explore water infrastructure impacts during the COVID-19 pandemic. Sustainable Cities and Society, 77, 103520. https://doi.org/10.1016/j.scs.2021.103520 DOI: https://doi.org/10.1016/j.scs.2021.103520
Ranjith, A., Vemavarapu, V. S., & Rao, L. (2025). Leak Detection in Urban Pipe Networks (pp. 565–578). https://doi.org/10.1007/978-981-97-7467-8_37 DOI: https://doi.org/10.1007/978-981-97-7467-8_37
Rapp, A. H., Capener, A. M., & Sowby, R. B. (2023). Adoption of Artificial Intelligence in Drinking Water Operations: A Survey of Progress in the United States. Journal of Water Resources Planning and Management, 149(7). https://doi.org/10.1061/JWRMD5.WRENG-5870 DOI: https://doi.org/10.1061/JWRMD5.WRENG-5870
Rego, S. A., Detenbeck, N. E., & Shen, X. (2024). EstuarySAT Database Development of Harmonized Remote Sensing and Water Quality Data for Tidal and Estuarine Systems. Water, 16(19), 2721. https://doi.org/10.3390/w16192721 DOI: https://doi.org/10.3390/w16192721
Renugadevi, R. (2025). An Optimal Method of Assessing the Quality of Water Using Integrated AI and Blockchain Methodology. In Smart Water Technology for Sustainable Management in Modern Cities (pp. 261–284). IGI Global. https://doi.org/10.4018/979-8-3693-8074-1.ch011 DOI: https://doi.org/10.4018/979-8-3693-8074-1.ch011
Sánchez Calle, J. E., Valles Coral, M. Á., & Gonzales Sánchez, P. A. (2021). Políticas promovedoras de la tecnificación y su efecto en la productividad acuícola. Ciencia & Tecnología Agropecuaria, 22(3), e2100. https://doi.org/10.21930/rcta.vol22_num3_art:2100 DOI: https://doi.org/10.21930/rcta.vol22_num3_art:2100
Shukla, A., Matharu, P. S., & Bhattacharya, B. (2023). Design and development of a continuous water quality monitoring buoy for health monitoring of river Ganga. Engineering Research Express, 5(4), 045073. https://doi.org/10.1088/2631-8695/ad0d40 DOI: https://doi.org/10.1088/2631-8695/ad0d40
Siraparapu, S. R., & Azad, S. M. A. K. (2024). Aqua-stream: an IoT based smart water management system for sustainable living. Indonesian Journal of Electrical Engineering and Computer Science, 36(3), 1460. https://doi.org/10.11591/ijeecs.v36.i3.pp1460-1469 DOI: https://doi.org/10.11591/ijeecs.v36.i3.pp1460-1469
Sörensen, J., Nilsson, E., Nilsson, D., Gröndahl, E., Rehn, D., & Giertz, T. (2024). Evaluation of ANN model for pipe status assessment in drinking water management. Water Supply, 24(5), 1985–1998. https://doi.org/10.2166/ws.2024.104 DOI: https://doi.org/10.2166/ws.2024.104
Stepanova, E., Orlov, V., Kukartsev, V., Pinchuk, I., & Suprun, E. (2024). RETRACTED: Machine learning approaches for water potability prediction: Addressing class imbalance with SMOTE. BIO Web of Conferences, 116, 03024. https://doi.org/10.1051/bioconf/202411603024 DOI: https://doi.org/10.1051/bioconf/202411603024
Strobl, R. O., Robillard, P. D., Shannon, R. D., Day, R. L., & McDonnell, A. J. (2006). A Water Quality Monitoring Network Design Methodology for the Selection of Critical Sampling Points: Part I. Environmental Monitoring and Assessment, 112(1–3), 137–158. https://doi.org/10.1007/s10661-006-0774-5 DOI: https://doi.org/10.1007/s10661-006-0774-5
Tao, H., Song, K., Wen, Z., Liu, G., Shang, Y., Fang, C., & Wang, Q. (2025). Remote sensing of total suspended matter of inland waters: Past, current status, and future directions. Ecological Informatics, 86, 103062. https://doi.org/10.1016/j.ecoinf.2025.103062 DOI: https://doi.org/10.1016/j.ecoinf.2025.103062
Tariq, R., Cetina-Quiñones, A. J., Cardoso-Fernández, V., Daniela-Abigail, H.-L., Soberanis, M. A. E., Bassam, A., & De Lille, M. V. (2021). Artificial intelligence assisted technoeconomic optimization scenarios of hybrid energy systems for water management of an isolated community. Sustainable Energy Technologies and Assessments, 48, 101561. https://doi.org/10.1016/j.seta.2021.101561 DOI: https://doi.org/10.1016/j.seta.2021.101561
Tasnuva, A., & Bari, Q. H. (2025). Employing the generalized Lasso model to evaluate key determinants of livelihood vulnerability in the Southwestern coastal Bangladesh. 020027. https://doi.org/10.1063/5.0249196 DOI: https://doi.org/10.1063/5.0249196
Timiraos, M., Díaz-Longueira, A., Michelena, Á., Zayas-Gato, F., Casteleiro-Roca, J.-L., & Calvo-Rolle, J. L. (2025). Potable Water Quality Assessment Through an Intelligent Classification Model (pp. 297–306). https://doi.org/10.1007/978-3-031-83117-1_28 DOI: https://doi.org/10.1007/978-3-031-83117-1_28
Uchimiya, M. (2024). Big data-driven water research towards metaverse. Water Science and Engineering, 17(2), 101–107. https://doi.org/10.1016/j.wse.2024.02.001 DOI: https://doi.org/10.1016/j.wse.2024.02.001
Xu, H., Berres, A., Liu, Y., Allen-Dumas, M. R., & Sanyal, J. (2022). An overview of visualization and visual analytics applications in water resources management. Environmental Modelling & Software, 153, 105396. https://doi.org/10.1016/j.envsoft.2022.105396 DOI: https://doi.org/10.1016/j.envsoft.2022.105396
Zeng, W., Do, N., Stephens, M., Cazzolato, B., & Lambert, M. (2025). Tracking Crack Development in Smart Water Networks Using IoT Acoustic Sensors. Journal of Water Resources Planning and Management, 151(1). https://doi.org/10.1061/JWRMD5.WRENG-6612 DOI: https://doi.org/10.1061/JWRMD5.WRENG-6612
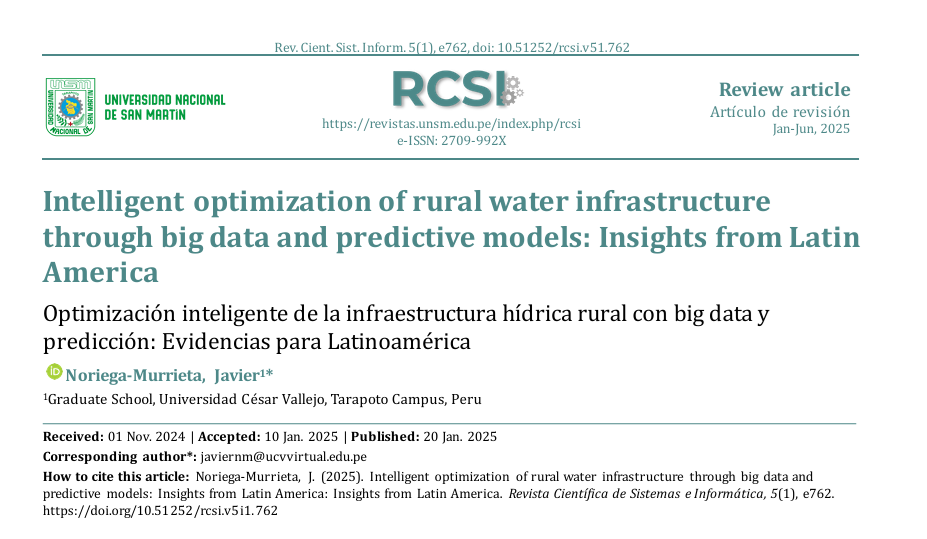
Downloads
Published
How to Cite
Issue
Section
License
Copyright (c) 2025 Javier Noriega-Murrieta

This work is licensed under a Creative Commons Attribution 4.0 International License.
The authors retain their rights:
a. The authors retain their trademark and patent rights, as well as any process or procedure described in the article.
b. The authors retain the right to share, copy, distribute, execute and publicly communicate the article published in the Revista Científica de Sistemas e Informática (RCSI) (for example, place it in an institutional repository or publish it in a book), with an acknowledgment of its initial publication in the RCSI.
c. Authors retain the right to make a subsequent publication of their work, to use the article or any part of it (for example: a compilation of their works, notes for conferences, thesis, or for a book), provided that they indicate the source of publication (authors of the work, journal, volume, number and date).