Application of the convolutional network Mask R-CNN for the estimation of the body weight of the guinea pig
DOI:
https://doi.org/10.51252/rcsi.v4i1.614Keywords:
mobile application, image dataset, artificial intelligence, convolutional networksAbstract
Artificial intelligence can contribute in tracking the productive cycle of the cuy through the application of convolutional networks, being a necessity the estimation of its weight. This study focused on the application of the Mask R-CNN convolutional network, using a mobile application as a tool for image capture. The methodology covered the following stages: i) bibliographic review, ii) data collection (images and pig weights), iii) image processing through data augmentation, iv) construction of a dataset (image selection and data transformation); , v) adaptation and training of the convolutional network, vi) analysis of the results to validate its performance, and finally, vii) implementation of a mobile application as a weight estimation tool. A set of 6244 pig images with their respective weights and masks was managed to be collected, together with the Mask R-CNN network adaptation. These tasks led to a correlation of R2 = 80.2% with the validation set, as well as to the development of a functional prototype capable of estimating the weight of pigs using the camera of a cell phone.
References
Abdulla, W. (2017). Mask R-CNN for object detection and instance segmentation on Keras and TensorFlow. GitHub. https://github.com/matterport/Mask_RCNN
Buayai, P., Piewthongngam, K., Leung, C. K., & Saikaew, K. R. (2019). Semi-Automatic Pig Weight Estimation Using Digital Image Analysis. Applied Engineering in Agriculture, 35(4), 521–534. https://doi.org/10.13031/aea.13084 DOI: https://doi.org/10.13031/aea.13084
Cang, Y., He, H., & Qiao, Y. (2019). An Intelligent Pig Weights Estimate Method Based on Deep Learning in Sow Stall Environments. IEEE Access, 7, 164867–164875. https://doi.org/10.1109/ACCESS.2019.2953099 DOI: https://doi.org/10.1109/ACCESS.2019.2953099
Cominotte, A., Fernandes, A. F. A., Dorea, J. R. R., Rosa, G. J. M., Ladeira, M. M., van Cleef, E. H. C. B., Pereira, G. L., Baldassini, W. A., & Machado Neto, O. R. (2020). Automated computer vision system to predict body weight and average daily gain in beef cattle during growing and finishing phases. Livestock Science, 232, 103904. https://doi.org/10.1016/j.livsci.2019.103904 DOI: https://doi.org/10.1016/j.livsci.2019.103904
Dohmen, R., Catal, C., & Liu, Q. (2022). Computer vision-based weight estimation of livestock: a systematic literature review. New Zealand Journal of Agricultural Research, 65(2–3), 227–247. https://doi.org/10.1080/00288233.2021.1876107 DOI: https://doi.org/10.1080/00288233.2021.1876107
Fernandes, A. F. A., Dórea, J. R. R., Fitzgerald, R., Herring, W., & Rosa, G. J. M. (2019). A novel automated system to acquire biometric and morphological measurements and predict body weight of pigs via 3D computer vision1. Journal of Animal Science, 97(1), 496–508. https://doi.org/10.1093/jas/sky418 DOI: https://doi.org/10.1093/jas/sky418
Gil Santos, V. (2007). Importancia del Cuy y su Competitividad en el Mercado. Archivos Latinoamericanos De Producción Animal, 15(5). https://ojs.alpa.uy/index.php/ojs_files/article/view/2741
González Marcos, A., Martínez de Pisón Ascacíbar, F., Pernía Espinoza, A., Alba Elías, F., Castejón Limas, M., Ordieres Meré, J., & Vergara González, E. (2006). Técnicas y Algoritmos Básicos de Visión Artificial (1st ed.). Universidad de La Rioja.
He, K., Gkioxari, G., Dollar, P., & Girshick, R. (2017). Mask R-CNN. 2017 IEEE International Conference on Computer Vision (ICCV), 2980–2988. https://doi.org/10.1109/ICCV.2017.322 DOI: https://doi.org/10.1109/ICCV.2017.322
Jensen, D., & Dominiak, K. (2018). Automatic estimation of slaughter pig live weight using convolutional neural networks. II International Conference on Agro BigData and Decision Support Systems in Agriculture.
Jun, K., Kim, S. J., & Ji, H. W. (2018). Estimating pig weights from images without constraint on posture and illumination. Computers and Electronics in Agriculture, 153, 169–176. https://doi.org/10.1016/j.compag.2018.08.006 DOI: https://doi.org/10.1016/j.compag.2018.08.006
Kashiha, M., Bahr, C., Ott, S., Moons, C. P. H., Niewold, T. A., Ödberg, F. O., & Berckmans, D. (2014). Automatic weight estimation of individual pigs using image analysis. Computers and Electronics in Agriculture, 107, 38–44. https://doi.org/10.1016/j.compag.2014.06.003 DOI: https://doi.org/10.1016/j.compag.2014.06.003
Konovalov, D. A., Saleh, A., Efremova, D. B., Domingos, J. A., & Jerry, D. R. (2019). Automatic Weight Estimation of Harvested Fish from Images. 2019 Digital Image Computing: Techniques and Applications (DICTA), 1–7. https://doi.org/10.1109/DICTA47822.2019.8945971 DOI: https://doi.org/10.1109/DICTA47822.2019.8945971
Lin, T.-Y., Maire, M., Belongie, S., Hays, J., Perona, P., Ramanan, D., Dollár, P., & Zitnick, C. L. (2014). Microsoft COCO: Common Objects in Context (pp. 740–755). https://doi.org/10.1007/978-3-319-10602-1_48 DOI: https://doi.org/10.1007/978-3-319-10602-1_48
Ma, W., Li, Q., Li, J., Ding, L., & Yu, Q. (2021). A method for weighing broiler chickens using improved amplitude-limiting filtering algorithm and BP neural networks. Information Processing in Agriculture, 8(2), 299–309. https://doi.org/10.1016/j.inpa.2020.07.001 DOI: https://doi.org/10.1016/j.inpa.2020.07.001
Miller, G. A., Hyslop, J. J., Barclay, D., Edwards, A., Thomson, W., & Duthie, C.-A. (2019). Using 3D Imaging and Machine Learning to Predict Liveweight and Carcass Characteristics of Live Finishing Beef Cattle. Frontiers in Sustainable Food Systems, 3. https://doi.org/10.3389/fsufs.2019.00030 DOI: https://doi.org/10.3389/fsufs.2019.00030
Mortensen, A. K., Lisouski, P., & Ahrendt, P. (2016). Weight prediction of broiler chickens using 3D computer vision. Computers and Electronics in Agriculture, 123, 319–326. https://doi.org/10.1016/j.compag.2016.03.011 DOI: https://doi.org/10.1016/j.compag.2016.03.011
Pezzuolo, A., Guarino, M., Sartori, L., González, L. A., & Marinello, F. (2018). On-barn pig weight estimation based on body measurements by a Kinect v1 depth camera. Computers and Electronics in Agriculture, 148, 29–36. https://doi.org/10.1016/j.compag.2018.03.003 DOI: https://doi.org/10.1016/j.compag.2018.03.003
Qiao, Y., Truman, M., & Sukkarieh, S. (2019). Cattle segmentation and contour extraction based on Mask R-CNN for precision livestock farming. Computers and Electronics in Agriculture, 165, 104958. https://doi.org/10.1016/j.compag.2019.104958 DOI: https://doi.org/10.1016/j.compag.2019.104958
Wang, Y., Yang, W., Winter, P., & Walker, L. (2008). Walk-through weighing of pigs using machine vision and an artificial neural network. Biosystems Engineering, 100(1), 117–125. https://doi.org/10.1016/j.biosystemseng.2007.08.008 DOI: https://doi.org/10.1016/j.biosystemseng.2007.08.008
Wilding-McBride, D., & Pun, D. (2018). Mask R-CNN utils. GitHub. https://github.com/DiUS/MaskRCNN-utils
Wongsriworaphon, A., Arnonkijpanich, B., & Pathumnakul, S. (2015). An approach based on digital image analysis to estimate the live weights of pigs in farm environments. Computers and Electronics in Agriculture, 115, 26–33. https://doi.org/10.1016/j.compag.2015.05.004 DOI: https://doi.org/10.1016/j.compag.2015.05.004
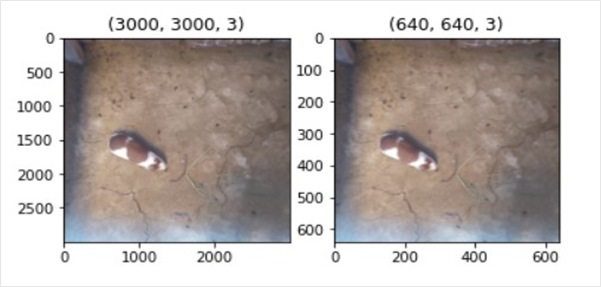
Published
How to Cite
Issue
Section
License
Copyright (c) 2024 Yeshica Isela Ormeño-Ayala, Abel Gabriel Zapata-Ttito

This work is licensed under a Creative Commons Attribution 4.0 International License.
The authors retain their rights:
a. The authors retain their trademark and patent rights, as well as any process or procedure described in the article.
b. The authors retain the right to share, copy, distribute, execute and publicly communicate the article published in the Revista Científica de Sistemas e Informática (RCSI) (for example, place it in an institutional repository or publish it in a book), with an acknowledgment of its initial publication in the RCSI.
c. Authors retain the right to make a subsequent publication of their work, to use the article or any part of it (for example: a compilation of their works, notes for conferences, thesis, or for a book), provided that they indicate the source of publication (authors of the work, journal, volume, number and date).