White line article recommendation system based on the KNN algorithm
DOI:
https://doi.org/10.51252/rcsi.v3i2.557Keywords:
e-commerce, Euclidean distance, K-neighbors, digital marketingAbstract
This research seeks to improve the digital marketing process for e-commerce issues, its main objective is to implement and operate a recommendation system that al-lows to correctly recommend a product to a customer saving time in their search and decision process. The K nearest neighbors’ algorithm and its Euclidean distance formula are used to improve the accuracy of the results. For this case we worked with the preferences of a user and a quantity of more than 100 products of different models and functionalities that are identified by identification variables such as color, brand, model, price, which are used to calculate the distance and generate "N" recommendations closer to the customer's tastes, the results show that the proposed algorithm is efficient in terms of product recommendation, generating recommendations efficiently in relation to customer preferences.
References
Adeniyi, D. A., Wei, Z., & Yongquan, Y. (2016). Automated web usage data mining and recommendation system using K-Nearest Neighbor (KNN) classification method. Applied Computing and Informatics, 12(1), 90–108. https://doi.org/10.1016/j.aci.2014.10.001
Afoudi, Y., Lazaar, M., & Al Achhab, M. (2021). Hybrid recommendation system combined content-based filtering and collaborative prediction using artificial neural network. Simulation Modelling Practice and Theory, 113, 102375. https://doi.org/10.1016/j.simpat.2021.102375
Bag, S., Ghadge, A., & Tiwari, M. K. (2019). An integrated recommender system for improved accuracy and aggregate diversity. Computers & Industrial Engineering, 130, 187–197. https://doi.org/10.1016/j.cie.2019.02.028
Cai, X., Hu, Z., Zhao, P., Zhang, W. S., & Chen, J. (2020). A hybrid recommendation system with many-objective evolutionary algorithm. Expert Systems with Applications, 159, 113648. https://doi.org/10.1016/j.eswa.2020.113648
Cao, B., Zhao, J., Liu, X., Kang, X., Yang, S., Kang, K., & Yu, M. (2018). Multiobjective recommendation optimization via utilizing distributed parallel algorithm. Future Generation Computer Systems, 86, 1259–1268. https://doi.org/10.1016/j.future.2017.09.005
Cao, Y., & Li, Y. (2007). An intelligent fuzzy-based recommendation system for consumer electronic products. Expert Systems with Applications, 33(1), 230–240. https://doi.org/10.1016/j.eswa.2006.04.012
Castro Gallardo, J. (2012). Un nuevo modelo ponderado para sistemas de recomendación basados en contenido con medidas de contingencia y entropía [Universidad de Jaén]. https://sinbad2.ujaen.es/sites/default/files/publications/TTII_JorgeCastro.pdf
Criado González, M. (2018). Análisis e implementación de un sistema de recomendación para la lista de la compra [Universidad Carlos III de Madrid]. http://hdl.handle.net/10016/2943
Franco Zapata, A. (2021). Sistemas de recomendación contextual [Universidad EAFIT]. http://hdl.handle.net/10784/3141
Guevara Albán, G. P., Guevara Albán, C., & Valverde, I. (2018). Sistemas de Recomendaciones: Una herramienta para mejorar la gestión de la información en las PYMES. Journal of Science and Research: Revista Ciencia e Investigación, 3(CITT2017), 121–127. https://doi.org/10.26910/issn.2528-8083vol3isscitt2017.2018pp121-127
Hssina, B., & Erritali, M. (2019). A personalized pedagogical objectives based on a genetic algorithm in an adaptive learning system. Procedia Computer Science, 151(2018), 1152–1157. https://doi.org/10.1016/j.procs.2019.04.164
Jain, A., Nagar, S., Singh, P. K., & Dhar, J. (2020). EMUCF: Enhanced multistage user-based collaborative filtering through non-linear similarity for recommendation systems. Expert Systems with Applications, 161, 113724. https://doi.org/10.1016/j.eswa.2020.113724
Karthik, R. V., & Ganapathy, S. (2021). A fuzzy recommendation system for predicting the customers interests using sentiment analysis and ontology in e-commerce. Applied Soft Computing, 108, 107396. https://doi.org/10.1016/j.asoc.2021.107396
Korus, K., Salamak, M., & Jasiński, M. (2021). Optimization of geometric parameters of arch bridges using visual programming FEM components and genetic algorithm. Engineering Structures, 241, 112465. https://doi.org/10.1016/j.engstruct.2021.112465
Kumar Sharma, A., Bajpai, B., Adhvaryu, R., Dhruvi Pankajkumar, S., Parthkumar Gordhanbhai, P., & Kumar, A. (2023). An Efficient Approach of Product Recommendation System using NLP Technique. Materials Today: Proceedings, 80, 3730–3743. https://doi.org/10.1016/j.matpr.2021.07.371
Li, M., Li, Y., Lou, W., & Chen, L. (2020). A hybrid recommendation system for Q&A documents. Expert Systems with Applications, 144, 113088. https://doi.org/10.1016/j.eswa.2019.113088
Liu, H., Zhao, J., Li, P., Zhao, P., & Wu, X. (2021). Shared-view and specific-view information extraction for recommendation. Expert Systems with Applications, 186, 115752. https://doi.org/10.1016/j.eswa.2021.115752
Luque-Ortiz, S. (2021). Estrategias de marketing digital utilizadas por empresas del retail deportivo. Revista CEA, 7(13), 0–22. https://doi.org/10.22430/24223182.1650
Marín López, J. C., & López Trujillo, M. (2020). Análisis de datos para el marketing digital emprendedor: Caso de estudio Parque de Innovación Empresarial - Universidad Nacional sede Manizales. Revista Universidad y Empresa, 22(38), 65. https://doi.org/10.12804/revistas.urosario.edu.co/empresa/a.7135
Martinez Rodriguez, J. R., & Alarcón Martínez, G. J. (2020). Análisis de la flexibilidad del proveedor y la participación de abastecimientos en el desempeño de manufactura para el sector de electrodomésticos (Analysis of supplier flexibility and purchasing participation in the manufacturing performance for applianc. Revista Innovaciones de Negocios, 17(33), 98–127. https://doi.org/10.29105/rinn17.33-6
Mlika, F., & Karoui, W. (2020). Proposed Model to Intelligent Recommendation System based on Markov Chains and Grouping of Genres. Procedia Computer Science, 176, 868–877. https://doi.org/10.1016/j.procs.2020.09.082
Ni, L., Lin, H., Zhang, M., & Zhang, J. (2018). Hybrid Filtrations Recommendation System based on Privacy Preserving in Edge Computing. Procedia Computer Science, 129, 407–409. https://doi.org/10.1016/j.procs.2018.03.016
Ravnik, J., Jovanovac, J., Trupej, A., Vištica, N., & Hriberšek, M. (2021). A sigmoid regression and artificial neural network models for day-ahead natural gas usage forecasting. Cleaner and Responsible Consumption, 3, 100040. https://doi.org/10.1016/j.clrc.2021.100040
Sánchez, N. (2019). Línea Blanca en auge, de la mano de la innovación y la eficiencia. Electromarket. https://www.electromarket.com/uploads/2019/08/linea_blanca_auge_19618_20190801014454.pdf
Ullal, M. S., Hawaldar, I. T., Soni, R., & Nadeem, M. (2021). The Role of Machine Learning in Digital Marketing. SAGE Open, 11(4), 215824402110503. https://doi.org/10.1177/21582440211050394
Uribe, C. I., & Sabogal Neira, D. F. (2021). Marketing digital en micro y pequeñas empresas de publicidad de Bogotá. Revista Universidad y Empresa, 23(40). https://doi.org/10.12804/revistas.urosario.edu.co/empresa/a.8730
Ye, G., & Zhao, X. (2018). Improved SVD algorithm based on Slope One. Proceedings of the 30th Chinese Control and Decision Conference, CCDC 2018, 1, 1002–1006. https://doi.org/10.1109/CCDC.2018.8407276
Zhang, F., Qi, S., Liu, Q., Mao, M., & Zeng, A. (2020). Alleviating the data sparsity problem of recommender systems by clustering nodes in bipartite networks. Expert Systems with Applications, 149, 113346. https://doi.org/10.1016/j.eswa.2020.113346
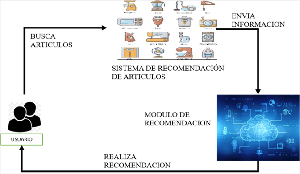
Published
How to Cite
Issue
Section
License
Copyright (c) 2023 Alexander Guevara-Fernandez, Marco A. Coral-Ygnacio

This work is licensed under a Creative Commons Attribution 4.0 International License.
The authors retain their rights:
a. The authors retain their trademark and patent rights, as well as any process or procedure described in the article.
b. The authors retain the right to share, copy, distribute, execute and publicly communicate the article published in the Revista Científica de Sistemas e Informática (RCSI) (for example, place it in an institutional repository or publish it in a book), with an acknowledgment of its initial publication in the RCSI.
c. Authors retain the right to make a subsequent publication of their work, to use the article or any part of it (for example: a compilation of their works, notes for conferences, thesis, or for a book), provided that they indicate the source of publication (authors of the work, journal, volume, number and date).