Evaluation of Snort and Suricata for detection of network probes and denial of service attacks
DOI:
https://doi.org/10.51252/rcsi.v2i2.363Keywords:
cybersecurity, pentest, performance, intrusion detection systemsAbstract
Intrusion detection systems are one of the most widely used tools to identify attacks or intrusions in data networks in order to ensure the confidentiality, availability and integrity of the information transmitted through them. Due to the complexity of its application in companies' cybersecurity schemes, it is necessary to carry out an objective evaluation of these solutions in order to select the tool that best suits the requirements of these organizations. The objective of this research is to quantitatively compare the performance of Snort and Suricata for the detection of network probes and denial of service attacks. The htop tool was used to test the performance of Snort and Suricata against network probes and denial of service attacks simulated with different Kali Linux applications. It was identified that Snort has a lower CPU consumption than Suricata during intrusion detection through signature analysis, however, Suricata showed better effectiveness rates. The results obtained contribute to decision making in relation to the selection, deployment and implementation of intrusion detection systems in business data networks.
References
Aludhilu, H., & Rodríguez-Puente, R. (2020). A Systematic Literature Review on Intrusion Detection Approaches. Revista Cubana de Ciencias Informáticas, 14(1), 58–78. http://scielo.sld.cu/scielo.php?pid=S2227-18992020000100058&script=sci_abstract&tlng=en
AlYousef, M. Y., & Abdelmajeed, N. T. (2019). Dynamically Detecting Security Threats and Updating a Signature-Based Intrusion Detection System’s Database. Procedia Computer Science, 159, 1507–1516. https://doi.org/10.1016/j.procs.2019.09.321
Arteaga Pucha, J. E. (2020). Evaluación de las funcionalidades de los sistemas de detección de intrusos basados en la red de plataformas open source utilizando la técnica de detección de anomalías. Latin-American Journal of Computing, 7(1), 49–64. https://doi.org/10.1016/j.procs.2019.09.321
Ashok, D., & Manikrao, V. (2015). Comparative study and analysis of network intrusion detection tools. International Conference on Applied and Theoretical Computing and Communication Technology. Davangere: IEEE, 312–315. https://doi.org/10.1109/ICATCCT.2015.7456901
Bijone, M. (2016). A Survey on Secure Network: Intrusion Detection & Prevention Approaches. American Journal of Information Systems, 4(3), 69–88. https://doi.org/10.12691/ajis-4-3-2
Bouziani, O., Benaboud, H., Chamkar, A. S., & Lazaar, S. (2019). A Comparative study of Open Source IDSs according to their Ability to Detect Attacks. 2nd International Conference on Networking, Information Systems & Security. Rabat: ACM, 1–5. https://doi.org/10.1145/3320326.3320383
Bustamante Garcia, S., Valles Coral, M. A., & Levano Rodriguez, D. (2020). Factores que contribuyen en la pérdida de información en las organizaciones. Revista Cubana de Ciencias Informáticas, 14(3), 148–165. https://rcci.uci.cu/?journal=rcci&page=article&op=view&path%5B%5D=1948&path%5B%5D=818
Caro Moreno, R. (2020). Despliegue y explotación de herramientas Open Source para la monitorización y gestión de eventos en un entorno virtualizado [Universidad de Cádiz]. http://hdl.handle.net/10498/23447
Divekar, A., Parekh, M., Savla, V., Mishra, R., & Shirole, M. (2018). Benchmarking datasets for Anomaly based Network Intrusion Detection: KDD CUP 99 alternatives. 3rd International Conference on Computing, Communication and Security (ICCCS), 1–8. https://doi.org/10.1109/CCCS.2018.8586840
Eset Security. (2021). Eset Security Report Latinoamérica 2021 (pp. 1–29). https://www.welivesecurity.com/wp-content/uploads/2021/06/ESET-security-report-LATAM2021.pdf
Fortinet. (2020). Threat Intelligence Insider Latin America 2020. https://www.fortinetthreatinsiderlat.com/en/Q2-2020/BR/html/trends#trends_position
Janampa Patilla, H., Huamani Santiago, H., & Meneses Conislla, Y. (2021). Snort Open Source como detección de intrusos para la seguridad de la infraestructura de red. Revista Cubana de Ciencias Informáticas, 15(3), 55–73. https://rcci.uci.cu/?journal=rcci&page=article&op=view&path%5B%5D=2042
Karim, I., Vien, Q.-T., Anh Le, T., & Mapp, G. (2017). A Comparative Experimental Design and Performance Analysis of Snort-Based Intrusion Detection System in Practical Computer Networks. Computers, 6(1). https://doi.org/10.3390/computers6010006
Kumar, D., & Singh, R. (2018). Comprehensive Review: Intrusion Detection System and Techniques. IOSR Journal of Computer Engineering, 18(4), 20–25. https://doi.org/10.9790/0661-1804032025
López-Avila, L., Acosta-Mendoza, N., & Gago-Alonso, A. (2020). Detección de anomalías basada en aprendizaje profundo: Revisión. Revista Cubana de Ciencias Informáticas, 13(3), 107–123. https://rcci.uci.cu/?journal=rcci&page=article&op=view&path%5B%5D=1874&path%5B%5D=779
Maciá-Fernández, G., Camacho, J., Magán-Carrión, R., Fuentes-García, M., García-Teodoro, P., & Theron, R. (2017). UGR’16: Un nuevo conjunto de datos para la evaluación de IDS de red. Jornadas de Ingeniería Telemática, 71–78. https://doi.org/10.4995/jitel2017.2017.6520
Maniriho, P., Mahoro, L. J., Niyigaba, E., Bizimana, Z., & Ahmad, T. (2020). Detecting Intrusions in Computer Network Traffic with Machine Learning Approaches. International Journal of Intelligent Engineering and Systems, 13(3), 433–445. https://doi.org/10.22266/IJIES2020.0630.39
Murphy, B. R. (2019). Comparing the performance of intrusion detection systems: snort and suricata [Colorado Technical University]. https://www.proquest.com/openview/885ab9a9d8f5c1b92d177780fbe81699/1?pq-origsite=gscholar&cbl=18750&diss=y
Olia Castellanos, L., & Milton García, B. (2020). Análisis y caracterización de conjuntos de datos para detección de intrusiones. Serie Científica de La Universidad de Las Ciencias Informáticas, 13(4), 39–52. https://publicaciones.uci.cu/index.php/serie/article/view/558
Park, W., & Ahn, S. (2017). Performance Comparison and Detection Analysis in Snort and Suricata Environment. Wireless Pers Commun, 94, 241–252. https://doi.org/10.1007/s11277-016-3209-9
Perdigón-Llanes, R. (2022). Suricata como detector de intrusos para la seguridad en redes de datos empresariales. Revista Ciencia UNEMI, 15(39), 44–53. https://doi.org/10.29076/issn.2528-7737vol15iss39.2022pp44-53p
Perdigón Llanes, R., & Orellana García, A. (2021). Sistemas para la detección de intrusiones en redes de datos de instituciones de salud. Revista Cubana de Informática Médica, 13(2). http://www.revinformatica.sld.cu/index.php/rcim/article/view/440
Perdigón Llanes, R., & Pérez Pino, T. M. (2020). Análisis holístico del impacto social de los negocios electrónicos en América Latina, de 2014 a 2019. Revista de Tecnología y Sociedad, 10(18). https://doi.org/10.32870/Pk.a10n18.459
Perdigón Llanes, R., & Ramírez Alonso, R. (2020). Plataformas de software libre para la virtualización de servidores en pequeñas y medianas empresas cubanas. Revista Cubana de Ciencias Informáticas, 14(1), 40–57. https://rcci.uci.cu/?journal=rcci&page=article&op=view&path%5B%5D=1901
Siddiqi, A. (2016). Performance Analysis of Open Source IDPS in Virtual Computing Environment [Northcentral University]. https://www.proquest.com/openview/decad1264757e8ac0c572441d0572fe9/1?cbl=18750&pq-origsite=gscholar
Solarte Martinez, G. R., Ocampo, C. A., & Castro Bermúdez, Y. V. (2017). Sistema de detección de intrusos en redes corporativas. Scientia et Technica, 22(1), 60–68. https://doi.org/10.22517/23447214.9105
Syed Ali Raza Shah, B. I. (2018). Performance comparison of intrusion detection systems and application of machine learning to Snort system. Future Generation Computer Systems, 80, 157–170. https://doi.org/10.1016/j.future.2017.10.016
Wang, X., Kordas, A., Hu, L., Gaedke, M., & Derrick. (2013). Administrative Evaluation of Intrusion Detection System. 2nd Annual Conference on Research in Information Technology, 47–52. https://doi.org/10.1145/2512209.2512216
World Economic Forum. (2020). The Global Risks Report 2020 (15th ed.). http://www3.weforum.org/docs/WEF_Global_Risk_Report_2020.pdf
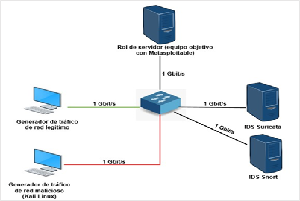
Published
How to Cite
Issue
Section
License
Copyright (c) 2022 Rudibel Perdigón-Llanes

This work is licensed under a Creative Commons Attribution 4.0 International License.
The authors retain their rights:
a. The authors retain their trademark and patent rights, as well as any process or procedure described in the article.
b. The authors retain the right to share, copy, distribute, execute and publicly communicate the article published in the Revista Científica de Sistemas e Informática (RCSI) (for example, place it in an institutional repository or publish it in a book), with an acknowledgment of its initial publication in the RCSI.
c. Authors retain the right to make a subsequent publication of their work, to use the article or any part of it (for example: a compilation of their works, notes for conferences, thesis, or for a book), provided that they indicate the source of publication (authors of the work, journal, volume, number and date).